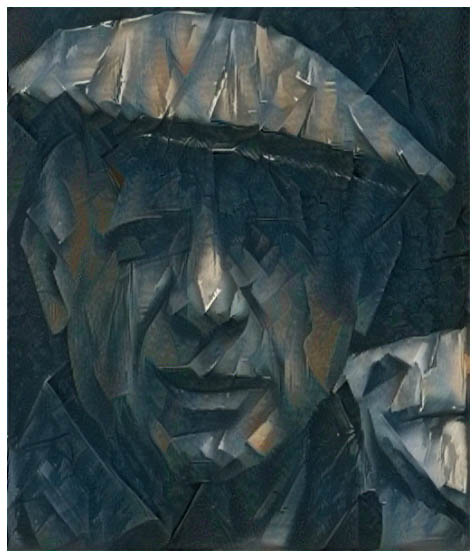
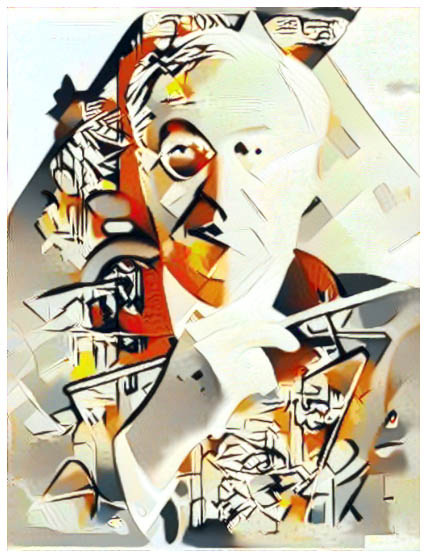
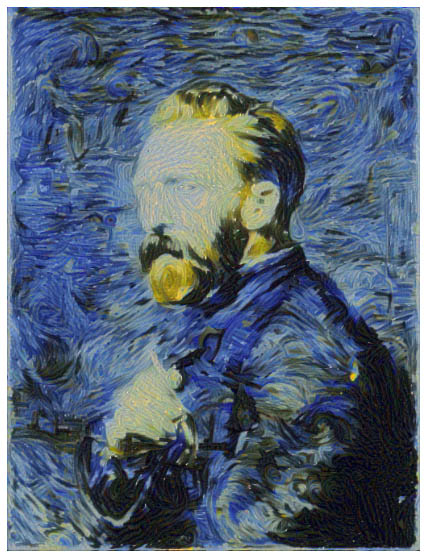
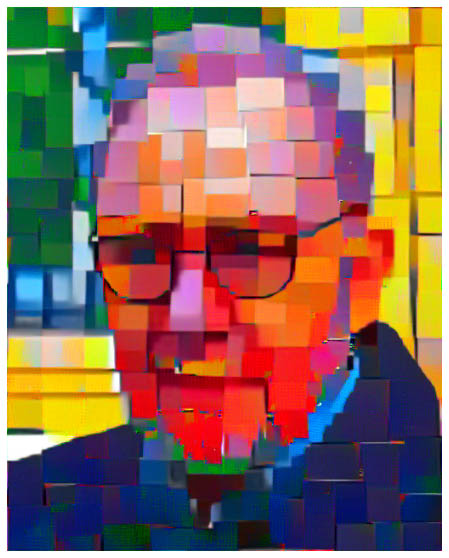
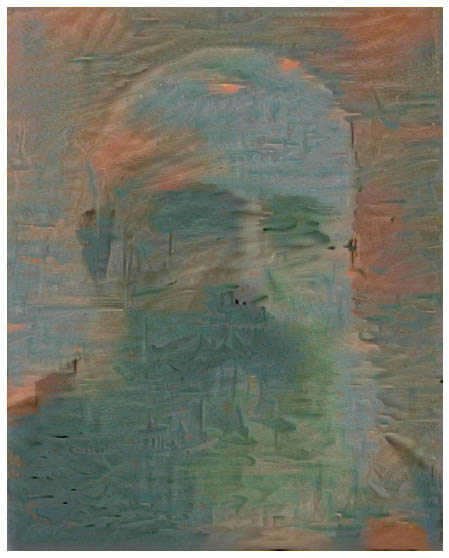
Style transfer aims to render the content of a given image in the graphical/artistic style of another image. The fundamental concept underlying Neural Style Transfer (NST) is to interpret style as a distribution in the feature space of a Convolutional Neural Network, such that a desired style can be achieved by matching its feature distribution. We show that most current implementations of that concept have important theoretical and practical limitations, as they only partially align the feature distributions. We propose a novel approach that matches the distributions more precisely, thus reproducing the desired style more faithfully, while still being computationally efficient. Specifically, we adapt the dual form of Central Moment Discrepancy (CMD), as recently proposed for domain adaptation, to minimize the difference between the target style and the feature distribution of the output image. The dual interpretation of this metric explicitly matches all higher-order centralized moments and is therefore a natural extension of existing NST methods that only take into account the first and second moments. Our experiments confirm that the strong theoretical properties also translate to visually better style transfer, and better disentangle style from semantic image content.
One fundamental idea in NST is to interpret style as a distribution in a feature space. In fact, most existing NST methods can be interpreted this way and has lead to a series of works all centered around aligning feature distributions of CNNs. However, existing style transfer methods suffer from rather elementary theoretical limitations. Broadly, there are two schools. Either the distributions are unrestricted, but the discrepancy between them is measured without adhering to the law of indiscernibles; or the distributions are approximated roughly with simple functions, so that they admit closed-form solutions.
Have a look at our paper for an in-depth theoretical analysis of existing methods and their limitations.
@article{kalischek2021light,
title={In the light of feature distributions: moment matching for Neural Style Transfer},
author={Nikolai Kalischek and Jan Dirk Wegner and Konrad Schindler},
year={2021},
eprint={2103.07208},
archivePrefix={arXiv},
primaryClass={cs.CV}
}